Lassa fever in Nigeria: lessons learnt
Back in March of this year we reached an important milestone in the collaboration between the Sabeti Lab and the Irrua Specialist Teaching Hospital (ISTH) in Edo State, Nigeria: we published a joint paper on the journal The Lancet Infectious Diseases describing the largest and most detailed retrospective cohort study of Lassa fever patients in Nigeria, and identifying the clinical and laboratory predictors of outcome observed at ISTH for this deadly disease. This is a culmination of several years of work, starting in 2013.
At the entrance of the Lassa ward at ISTH with Christopher Iruolagbe, one of the clinicians.
First of all, I will give a brief introduction to Lassa fever. This is a member of a family of diseases called Viral Hemorrhagic Fevers (VHFs), which includes Ebola, dengue, and yellow fever among many others. These illnesses are caused by different viruses, but all have fever and hemorrhage as clinical manifestations. The 2014-2016 Ebola outbreak caused widespread concern due to its high mortality and fear for a worldwide pandemic, although its spread was largely contained to the African nations of Liberia, Sierra Leone, and Guinea. The outbreak left a large impact in the region, with almost 30,000 total cases and over 15,000 deaths. In contrast, Lassa fever is an endemic disease, with cases occurring throughout the year in West Africa, and presenting a wide range of clinical severity: most people don’t get sick enough to go to the hospital, but a small percentage become acutely ill and need medical attention. It is estimated that 300,000 people get infected with the Lassa virus every year, but less than 5% of those end up going to the hospital. The overall mortality among hospitalized cases is around 20%, lower than Ebola, but it can be much higher than that for elderly patients and pregnant women. Another difference with Ebola is the natural host of the virus: in the case of Lassa fever, it is the mastomys rat, which enters into people’s homes looking for food and spreads the virus through feces and urine, while for Ebola it is likely to be fruit bats instead. Even though the pathogens causing these diseases were discovered only in the last 50 years (Lassa fever in 1969, Ebola in 1976), genetic studies from our lab indicate that Lassa fever is an old disease, with the virus spreading out of Nigeria at least 400 years ago. A similar picture appears for Ebola, leading to the question of whether we are in the presence of emerging diseases or diagnoses.
TEM micrograph of Lassa virus virions.
Back in 2013, I just began developing the visualization tool Mirador at Fathom Information Design, and was looking for “real world” datasets to apply Mirador to. Around that time, Dr Peter Okokhere, the head of the Lassa fever ward at ISTH, was visiting the Sabeti Lab, and had compiled the records of all the patients treated in the ward since 2011 into an anonymized Excel spreadsheet. Mirador was designed precisely to handle that kind of tabular data, so it seemed to us that it should be straightforward to use Mirador to carry out exploratory analysis of Dr Okokhere’s dataset. In particular, we were interested in finding correlations between the different demographic, clinical, and laboratory variables collected for all patients and their outcome (death or survival). A subsequent step was to apply Machine Learning in order to train prognostic models that could eventually be helpful for clinicians, for example to triage patients upon admission to the ward depending on their death risk (so that time and material resources would be prioritized for high-risk patients). The models’ predictions could also identify the clinical features most strongly the risk, and hence inform medical judgment.
Mirador displaying ISTH data.
However, the work on the ISTH clinical data had to be put on hold as the lab shifted its focus and resources to the Ebola outbreak during the next two years. Some of the initial modeling approaches we were developing for the ISTH dataset were applied to similar datasets from Ebola patients, and this work led to some publications (here and here), where we investigated the possibility of deploying this prognostic models to the clinic in the form of medical apps for patient triage, care, and management. By late 2015, the Ebola outbreak was beginning to subside, and we were able to come back to our research on Lassa. In the meantime, Dr Okokhere had incorporated patients treated in 2014 and 2015, which enlarged the dataset to nearly 300 patients. At that time, I started to realize that I was originally too enthusiastic about the applicability of prognostic models to inform clinical decisions. As I learned while after delving deeper into the topic, such models need to be trained on much larger cohorts and then validated on independently-obtained datasets, so that they can be generalized to new patients. Widely used prognostic scores such as APACHE II or the Framingham Risk Score took thousands of patients and many years to be developed. As unique and detailed as the ISTH dataset is, it cannot support the creation of a Lassa fever prognostic score yet. Thanks to Dr Okokhere’s efforts to digitalize the paper medical records of the patients treated in the ward, we were able to have some data, but unfortunately there are no similar datasets available for analysis and validation. Only one other study, published in 1987, included more than 300 confirmed cases of Lassa fever and detailed demographic, clinical, and laboratory data from patients in Sierra Leone between 1977 and 1979.
Regardless of the current limitations, the ISTH dataset allowed us to provide a significant update on the clinical knowledge of Lassa fever in Nigeria, and to find the most important manifestations of the disease among the patients treated at ISTH.
Map of cases treated at ISTH between 2011 and 2015, clustered by geographical location and shaded by case fatality rate.
The predictive models also proved to be very useful, not as prognostic scores, but to test the independence between various biomarkers that characterize the pathophysiology of the disease. This, in addition other complementary results and previous experience from the clinicians at ISTH, led us to make hypothesis of medical relevance (e.g.: Lassa virus may damage the kidney cells in some of the patients), which we need to explore further.
Predictive performance of the logistic regression model trained on the ISTH data. The horizontal axis shows the mortality risk threshold used to predict death vs survival, and the right vertical axis the corresponding sensitivity and specificity of the model. The bars indicate the actual number of patients and mortality within each risk bin.
However, one of the requirements to move forward with this research and also improve patient care is to build better on-site capacity for data collection and management. This not only impacts the handling of the patients’ medical records, but also the laboratory samples that are used for diagnosis, clinical decision, and research. For this reason, we have been designing mobile apps for collecting clinical and laboratory data, using Dimagi’s CommCare platform. This platform has enabled us to translate paper-based protocols for data collection into digital forms that can work with limited internet connectivity, store the records in a HIPPA-compliant database, and generate real-time reports. These apps are currently undergoing the final stages of debug and testing, and will soon be deployed at ISTH’s Lassa ward and research lab for daily use.
CommCare clinical app for patient management.
As part of the testing process, I had the chance to visit ISTH recently, together with my lab colleague, Dr Kayla Barnes. We worked with the clinicians and lab staff, going through all the data entry modules in the apps and making sure that they function as expected and fit the workflow patient and sample management protocols. Being my first time in Nigeria and in Africa in general, this was an exceptional experience for me, and felt incredibly welcomed by all people we met during the visit. We even got tailor-made traditional Nigerian dresses we wore the last day of our stay at ISTH, as you can see in some of the pictures below!
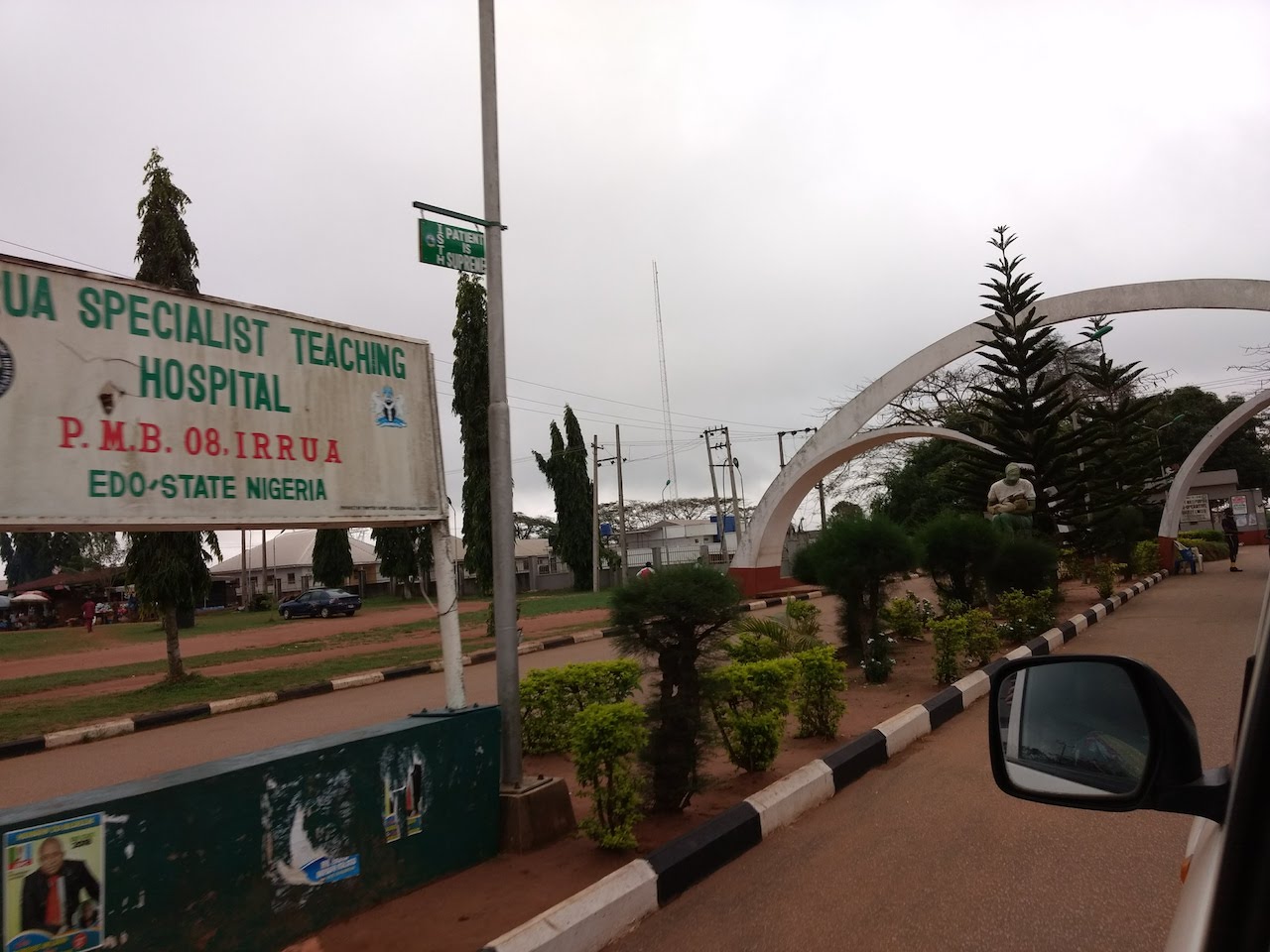

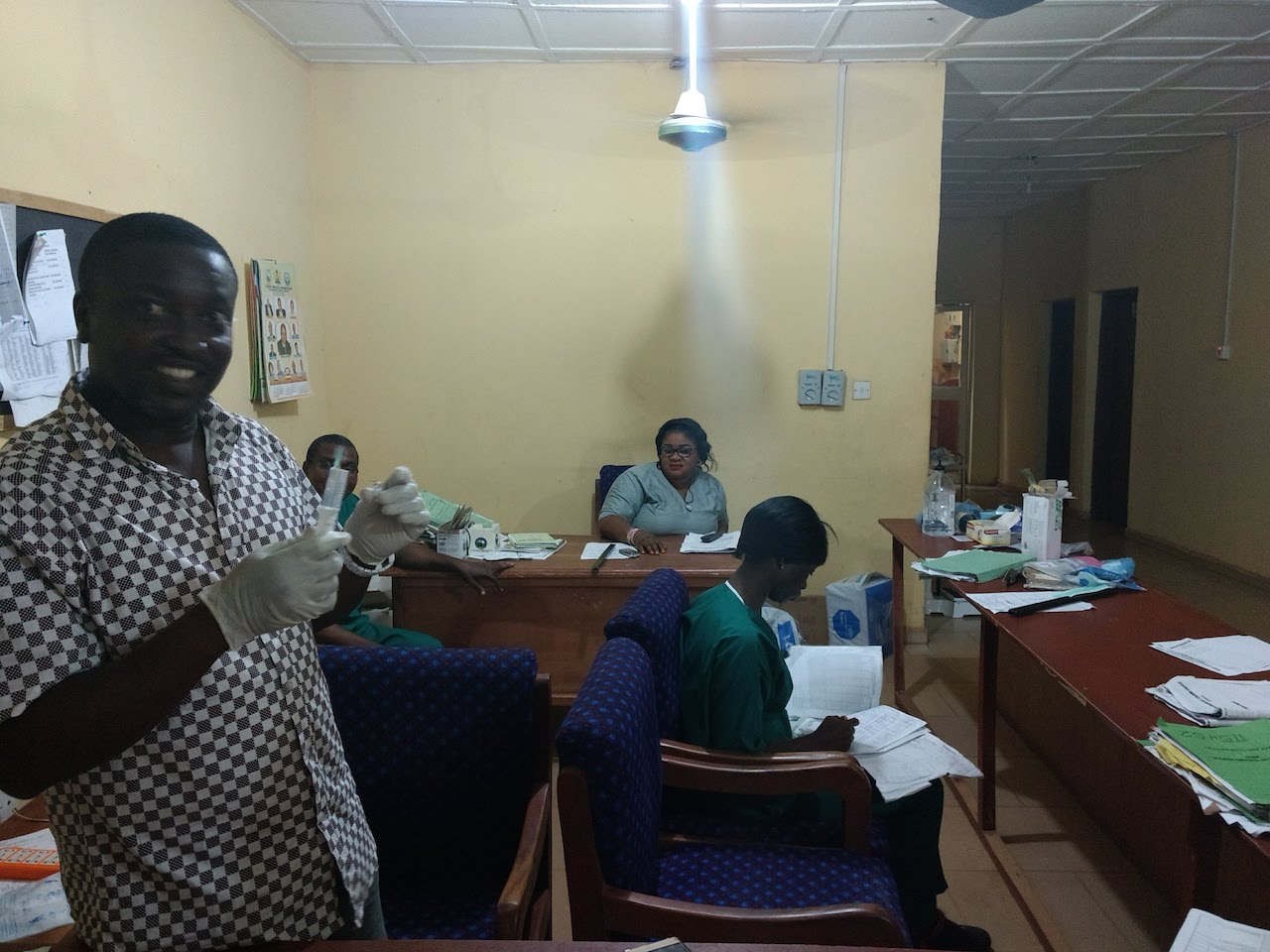
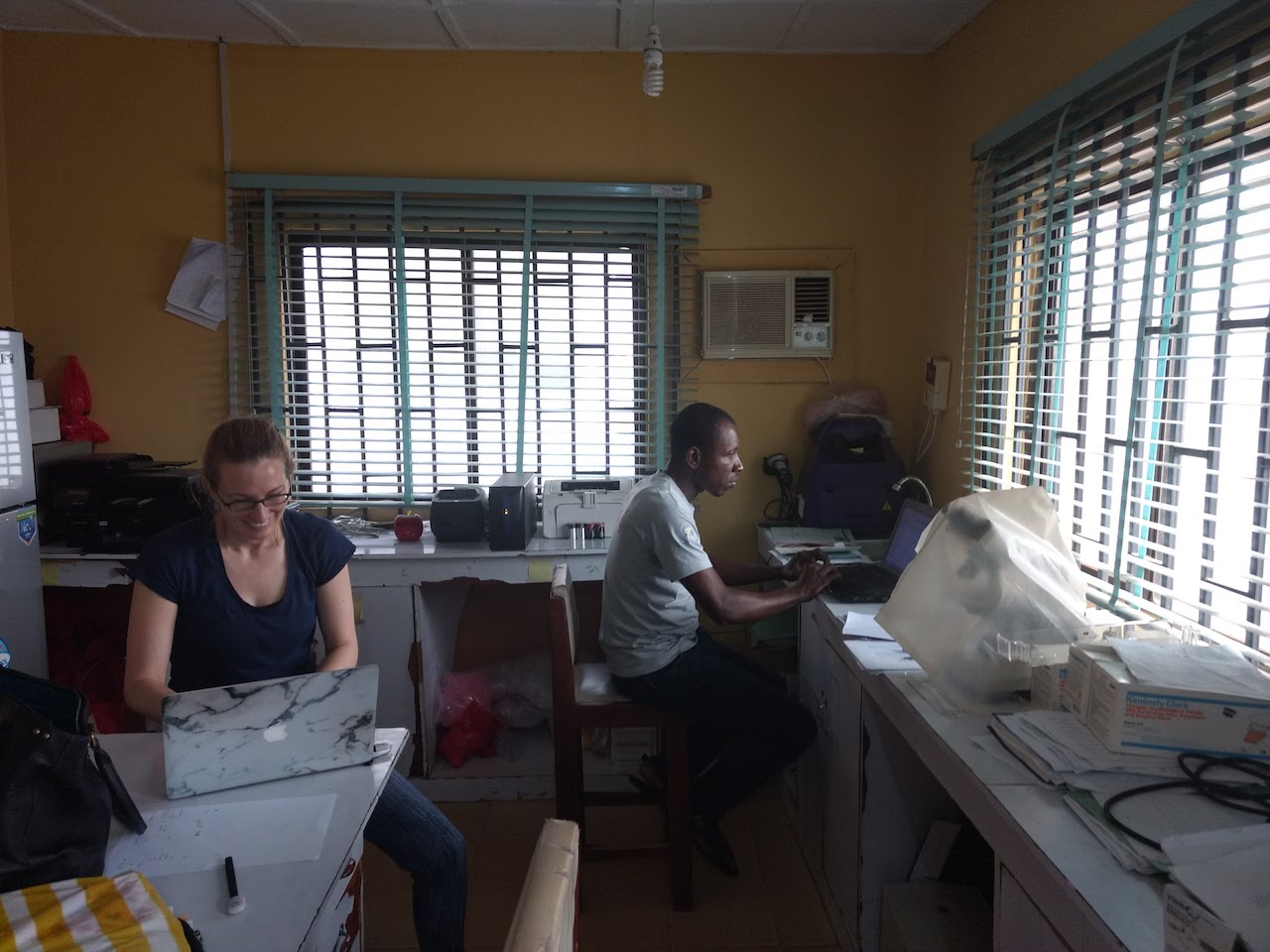

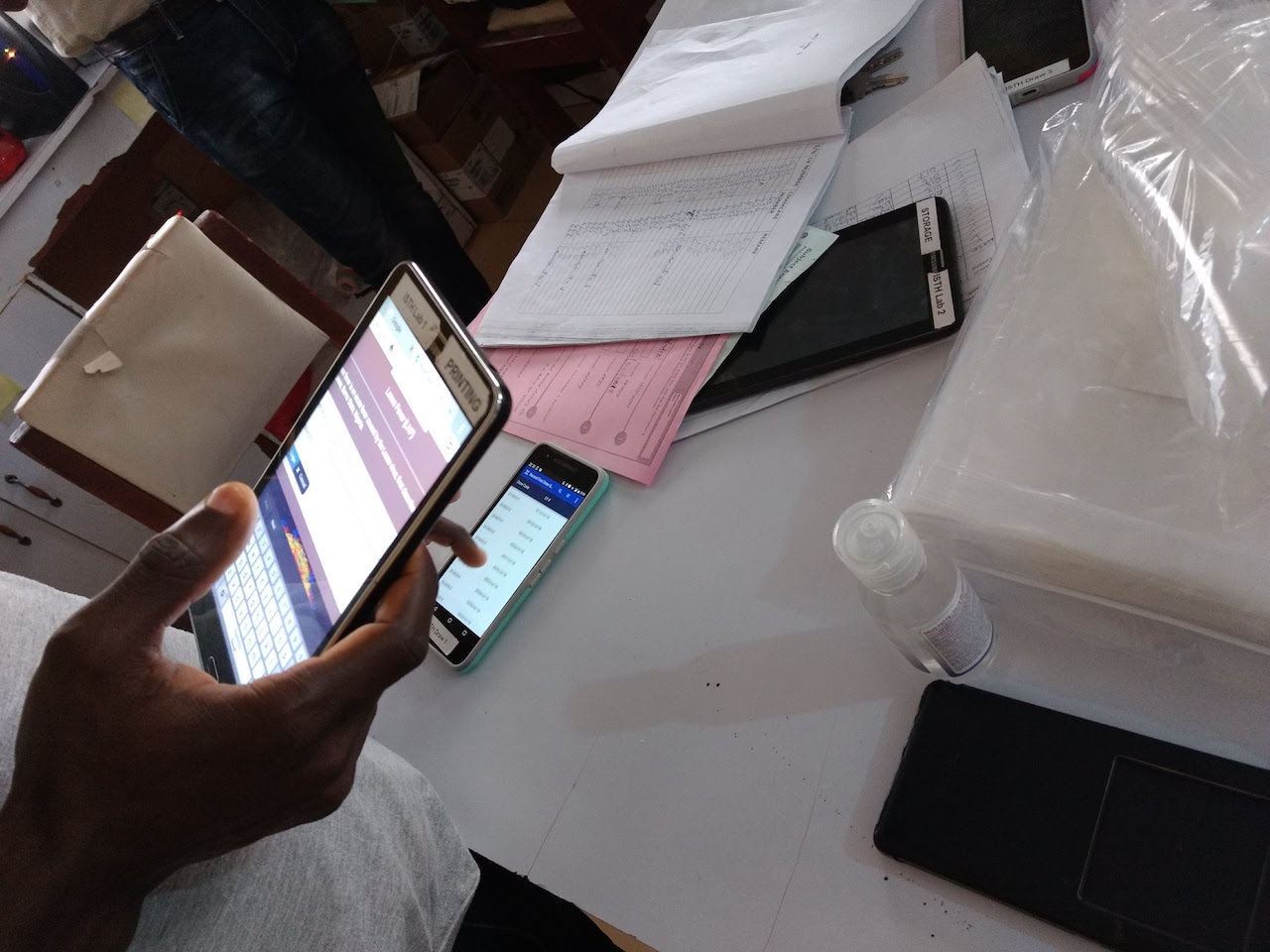
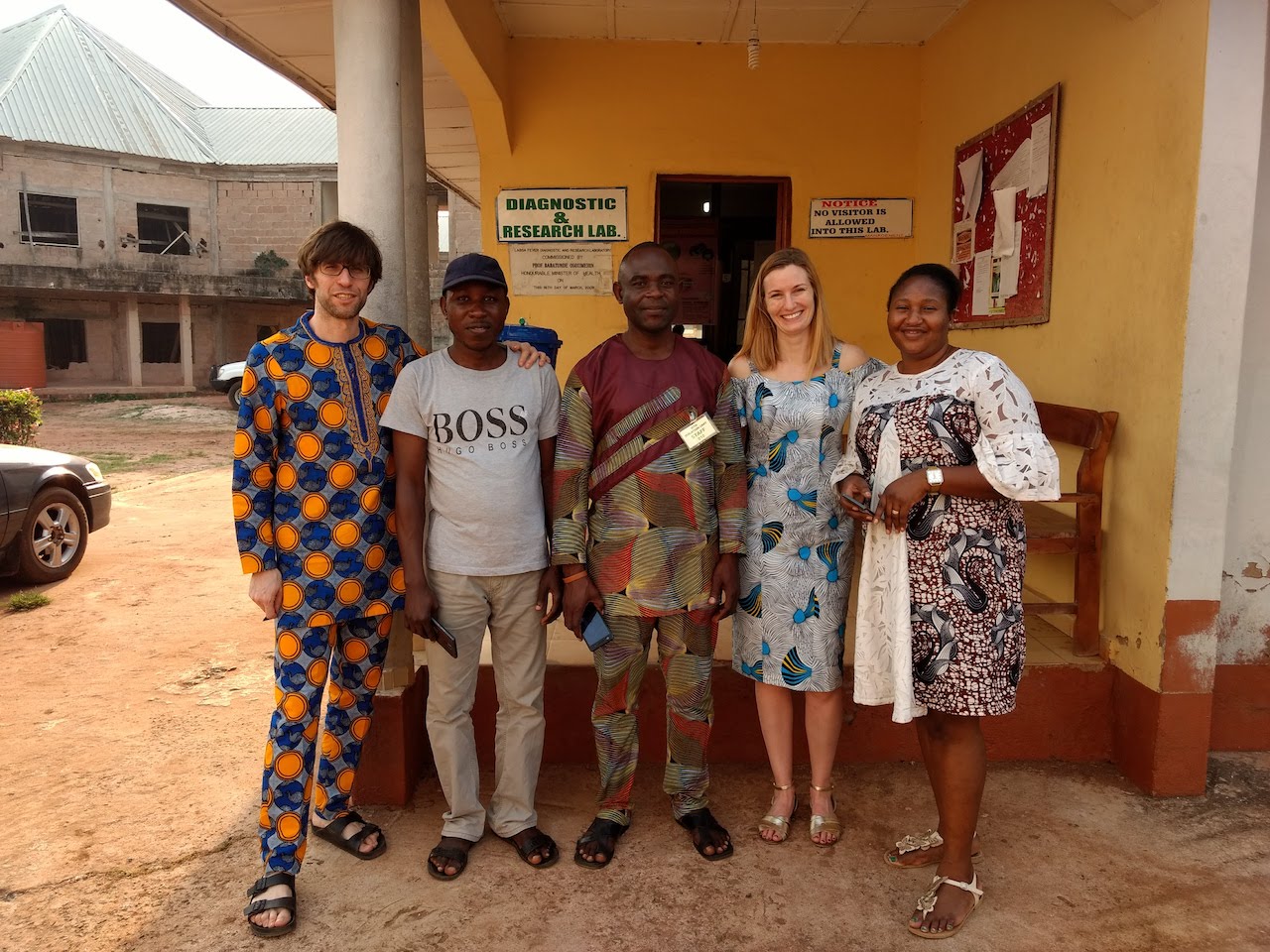
Some pictures from our trip to ISTH in March 2018.
As much as we enjoyed a very successful visit and the hospitality of our Nigerian hosts, Nigeria and ISTH in particular were still recovering of the largest recorded Lassa fever outbreak in history: more than 200 confirmed cases were received and tested at ISTH between January and February of this year, which is more than all the cases from 2016 and 2017 combined. This outbreak was covered by several news sources, and it put a lot of strain on the Lassa ward personnel as they run out of beds and had to resort to temporary spaces to accommodate all patients. Fortunately, by the time we arrived the situation was gradually coming back to normal, as reported by the Nigerian Center for Disease Control. However, this recent event is a reminder of the threat posed by Lassa fever and other emerging infectious diseases, and how we need efficient detection and containment mechanisms to stop outbreaks and avoid loss of human life.
Research continues at ISTH and we hope to find insights about the recent outbreak from the viral sequences generated in Nigeria, thanks to the capacity building efforts from the African Center of Excellence for Genomics of Infectious Diseases, which our lab is part of together with several African and international partners. Among further next steps, we plan to carry out analyses of the combined the genomic and clinical data that could shed light on the genetic origin for the variability in the clinical manifestation of Lassa fever, and to develop new privacy-preserving Machine Learning algorithms that would allow us to respond to an outbreak faster by sharing data and models in real-time while protecting patient’s privacy.
comments powered by Disqus